Quantum Annealing Breakthrough: Quantum Computer Outperforms Fastest Supercomputers
Quantum Annealing Breakthrough: Quantum Computer Outperforms Fastest Supercomputers
May 18, 2025
In a major milestone for quantum computing, researchers Humberto Muñoz-Bauza and Daniel Lidar have demonstrated the first clear scaling advantage of quantum annealing in approximate optimization—a critical task in fields like logistics, finance, and machine learning. Published in Physical Review Letters, the study reveals that quantum annealing can outperform the best-known classical heuristic method in solving complex optimization problems, provided certain conditions are met.
The Challenge: Optimization at Scale
Many real-world problems—from routing delivery trucks to portfolio optimization—boil down to finding the best configuration among a massive number of possibilities. These problems often involve “spin glasses,” which are notoriously difficult to solve. Quantum annealing, a method that leverages quantum mechanics to search for low-energy (optimal) configurations, has long been a candidate for outperforming classical algorithms in these scenarios.
But until now, no study had definitively shown a scaling advantage—meaning that as problem size increases, quantum annealers solve problems more efficiently than classical methods in a measurable way.
Quantum Annealing Correction (QAC): A Key Innovation
The study’s breakthrough lies in the use of Quantum Annealing Correction (QAC), a method that applies error suppression by embedding error-correcting codes directly into the quantum annealer's architecture. QAC leverages a majority-voting scheme among physical qubits to protect logical qubit states, effectively reducing the impact of hardware noise and analog errors.
Using QAC, the researchers implemented a highly error-tolerant system on the D-Wave Advantage quantum annealer. They tested it on large-scale spin-glass problems using up to 1,322 logical, error-corrected qubits—the largest such demonstration to date.
The Benchmark: Outperforming the Best Classical Heuristic
The quantum system was pitted against parallel tempering with isoenergetic cluster moves (PT-ICM), a top-tier classical algorithm. The key metric was time-to-epsilon (TTε), which measures how quickly a solver finds solutions within a certain percentage (ε) of the optimal answer.
At an optimality gap of 1% or higher, quantum annealing with QAC consistently outscaled PT-ICM, providing faster approximate solutions as problem sizes grew.
Even without error correction (a method called C3), quantum annealers showed some advantage, though QAC clearly performed best.
This scaling advantage was not just a one-time performance win—it persisted across varying problem sizes and noise levels, and the improvement grew stronger with larger optimality gaps.
Key Findings
QAC shows better scaling than classical methods at approximate solutions (ε ≥ 1.0%), which could translate to real-world speedups in tasks like machine learning or supply chain management.
Error correction is critical—without QAC, performance drops noticeably.
Fast annealing schedules (coherent regimes) do not necessarily improve scaling and may be more susceptible to thermal errors.
Quantum tunneling is suspected to play a role in this speedup, offering a potential mechanism behind the improved performance.
Why This Matters
This research marks a turning point in quantum optimization, providing the first evidence that quantum annealers can surpass classical algorithms not just in runtime but in how they scale with increasing problem complexity.
While this is an early-stage result confined to synthetic spin-glass problems, it opens the door to applying quantum advantage in real-world use cases, particularly where high-precision, approximate answers are acceptable or even preferred.
What’s Next?
The next challenge is to:
Extend these findings to more complex, application-specific problems with dense connectivity.
Optimize hardware schedules and error-suppression techniques for broader use.
Continue refining metrics like TTε to evaluate future quantum and hybrid solvers.
Bottom Line:
Quantum computing has taken a significant leap from theory to tangible performance gains. Thanks to innovations like QAC and improved benchmarking, we now have credible evidence that quantum annealing isn’t just a niche tool—but a competitive and scalable approach for solving some of the world’s hardest computational problems.
Citation: Muñoz-Bauza, H., & Lidar, D. (2025). Scaling Advantage in Approximate Optimization with Quantum Annealing. Physical Review Letters, 134(16), 160601. https://doi.org/10.1103/PhysRevLett.134.160601
May 18, 2025
Quantum Insights



Texas Quantum Initiative Passes: Lone Star State Bids to Become Quantum Powerhouse
Jul 10, 2025



Europe’s Quantum Surge: Bridging the Private Funding Gap for Tech Dominance
Jul 8, 2025



Racing the Quantum Threat: 5 Nations Compress Post-Quantum Cryptography Timelines
Jun 26, 2025



Microsoft’s Azure Quantum Unveils 4D Code Plan to Tame Quantum Errors
Jun 22, 2025



How Post‑Quantum Cryptography Could Have Stopped the $1.5 Billion Bybit Hack



IIT Delhi Achieves Quantum Breakthrough: Wireless Communication Over 1 Kilometer
Jun 18, 2025



Caltech Scientists Achieve Hyper-Entanglement in Atomic Motion: A Quantum Leap in Control and Coherence
Jun 17, 2025



Quantum Boom: Surge in Tech Deals & Funding Marks a Turning Point in 2025
Jun 15, 2025



Pasqal Charts Bold Course: Roadmap to 10,000 Qubits and Fault-Tolerant Quantum Computing
Jun 12, 2025



Quantum at a Turning Point: Nvidia CEO Declares Industry at ‘Inflection Point’
Jun 11, 2024



IBM Unveils Next-Generation Quantum Processor, Ushering In a New Era of Computation
Jun 10, 2025



IonQ Acquires Oxford Ionics for $1.08 Billion: A Bold Leap Toward Fault‑Tolerant Quantum Computing
Jun 9, 2025



Post‑Quantum Cryptography Takes Center Stage at Infosecurity Europe 2025
Jun 7, 2025



Oxford Quantum Circuits Unveils Bold Roadmap to 50,000 Logical Qubits by 2034
Jun 5, 2025



Caltech Achieves Hyper-Entanglement: A Quantum Breakthrough with Major Implications
Jun 5, 2025



Massive Data Breach Exposes Apple ID Logins: Why Post-Quantum Cryptography Must Start Now
Jun 4, 2025



A Quantum Celebration: UN Declares 2025 the International Year of Quantum Science and Technology
Jun 4, 2025



Crypto Asset Manager Grayscale Eyes the Quantum Frontier with Proposed Quantum Computing ETF
Jun 3, 2025



Quantum Entanglement: The Spooky Phenomenon That Could Transform Technology
Jun 2, 2025



Colt, Honeywell, and Nokia Launch Space-Based Trial for Quantum-Safe Cryptography
Jun 2, 2025



Surrogate Models Take Center Stage: A Smarter Way to Optimize Quantum Networks
May 31, 2025



Securing the Internet of Things: Why Post-Quantum Cryptography Is Critical for IoT's Future
May 30, 2025



Nord Quantique’s Multimode Qubit Breakthrough: A Leap Toward Scalable Quantum Computing
May 30, 2025



The 2025 Retail Cyberstorm: How Post-Quantum Cryptography Could Have Prevented Major Breaches
May 29, 2025



Microsoft’s Quantum Leap: Inside the Majorana Chip That Could Revolutionize Computing
May 29, 2025



Should Post-Quantum Cryptography Start Now? The Clock Is Ticking
May 28, 2025



Cracking RSA with Fewer Qubits: What Google's New Quantum Factoring Estimate Means for Cybersecurity
May 28, 2025



Quantum Arms Race: U.S. Defense Intelligence Flags Rivals’ Growing Military Use of Quantum Tech
May 27, 2025



Quantum Threats and Bitcoin: Why BlackRock’s Warning Matters for the Future of Crypto Security
May 27, 2025



Sudbury's SNOLAB Ventures into Quantum Computing Research
May 26, 2025



Lockheed Martin and IBM Pioneer Quantum-Classical Hybrid Computing for Complex Molecular Simulations
May 23, 2025



Why the Moon Matters for Quantum Computing: From Helium-3 to Off-Planet Quantum Networks
May 23, 2025



NIST Approves Three Post-Quantum Cryptography Standards: A Milestone for Digital Security
May 22, 2025



Scientists Connect Quantum Processors via Fiber Optic Cable for the First Time
May 21, 2025



Quantum Computing and Encryption Breakthroughs in 2025: A New Era of Innovation
May 21, 2025



How CISOs Can Defend Against the “Harvest Now, Decrypt Later” Threat
May 20, 2025



NVIDIA Expands Quantum and AI Ecosystem in Taiwan Through Strategic Partnerships and Supercomputing Initiatives
May 19, 2025



Quantum Annealing Breakthrough: Quantum Computer Outperforms Fastest Supercomputers
May 18, 2025



Quantum Computing's New Frontier: How the $1.4 Trillion US–UAE Investment Deal is Shaping the Industry
May 16, 2025



Quantum Computing Meets Cancer Research: A New Frontier in Drug Discovery
May 16, 2025



Quantum Industry Leaders Urge Congress to Reauthorize and Expand National Quantum Initiative
May 15, 2025



Honeywell's Quantinuum and Qatar's Al Rabban Capital Forge $1 Billion Quantum Computing Joint Venture
May 15, 2025



Advancing Quantum Machine Learning with Multi-Chip Ensemble Architectures
May 14, 2025



How will the new US-Saudi Arabia AI deal effect the Quantum Computing industry?
May 14, 2025



Saudi Arabia's $600 Billion AI Push: Amazon, Nvidia, and Global Tech Giants Lead the Charge
May 14, 2025



Quantum Computing Breakthrough: Diamond Qubits Achieve Unprecedented Precision
Apr 28, 2025



Australia’s Quantum Cryptography Roadmap: Preparing for a Post-Quantum Future
Apr 26, 2025
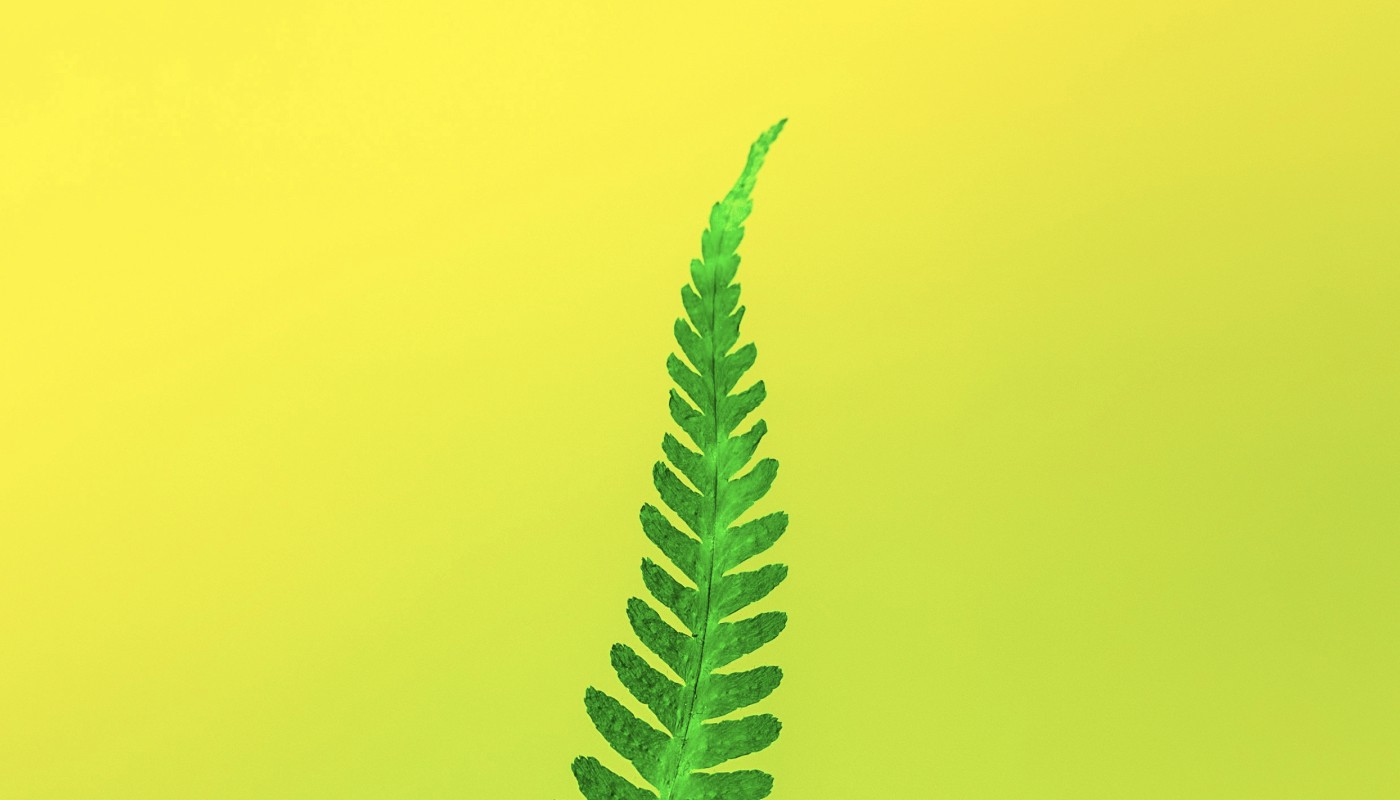
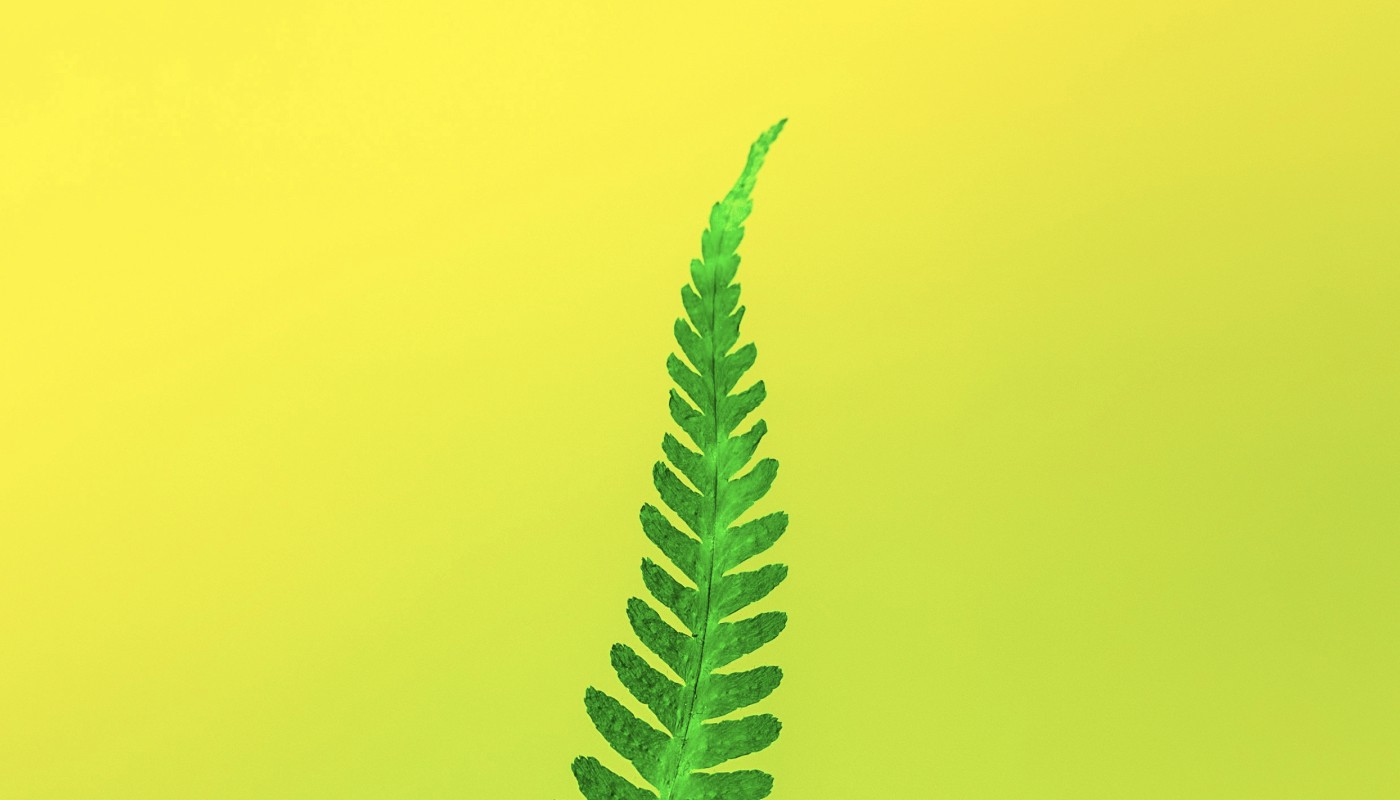
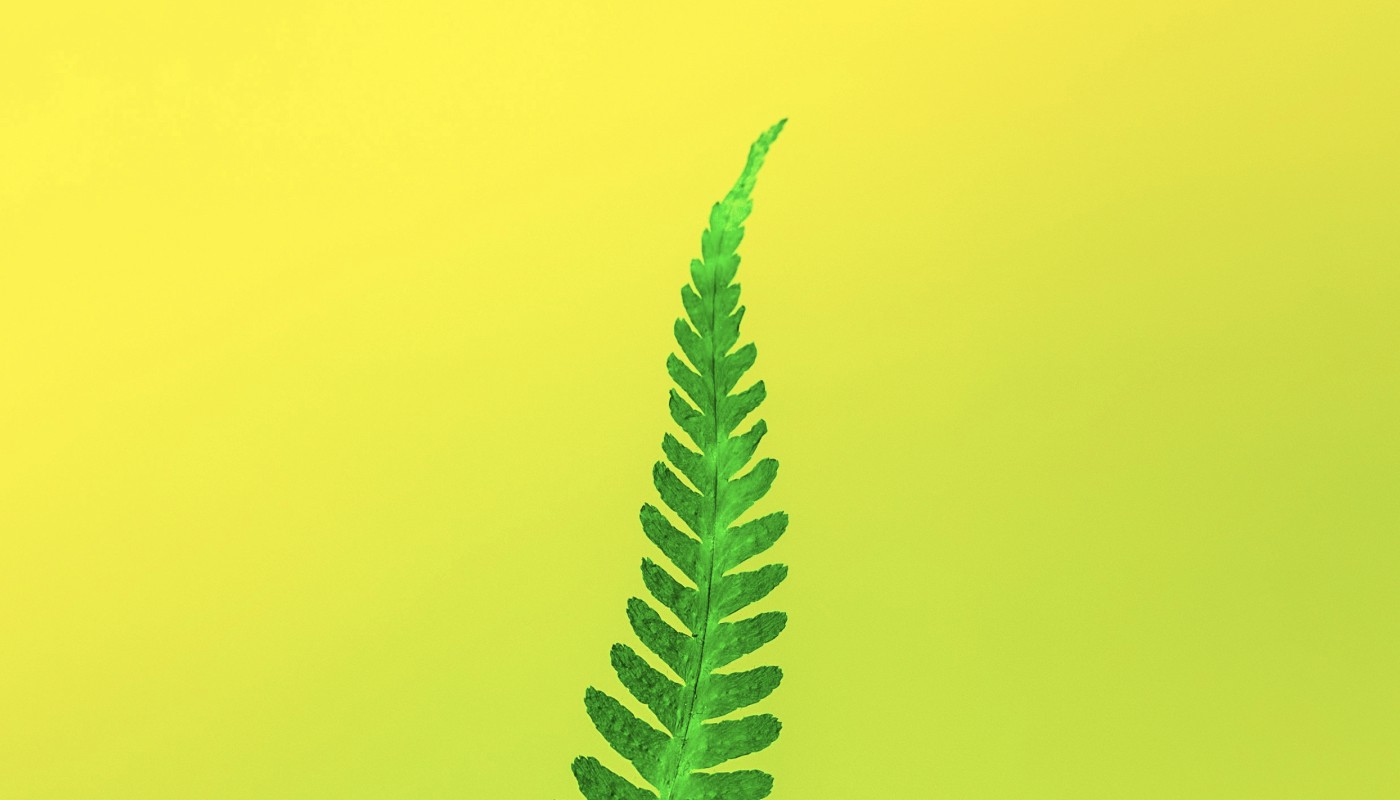
Harvest Now, Decrypt later
Apr 25, 2025
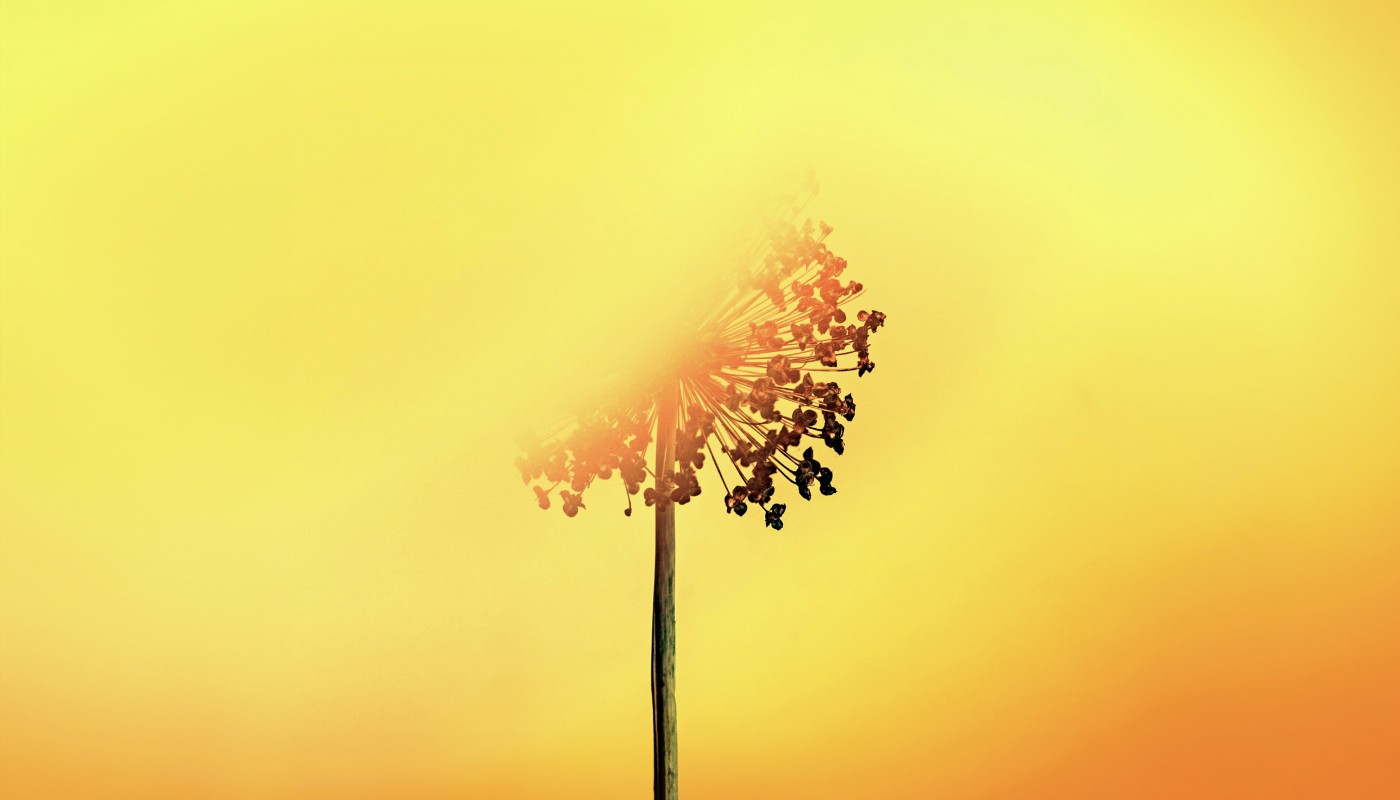
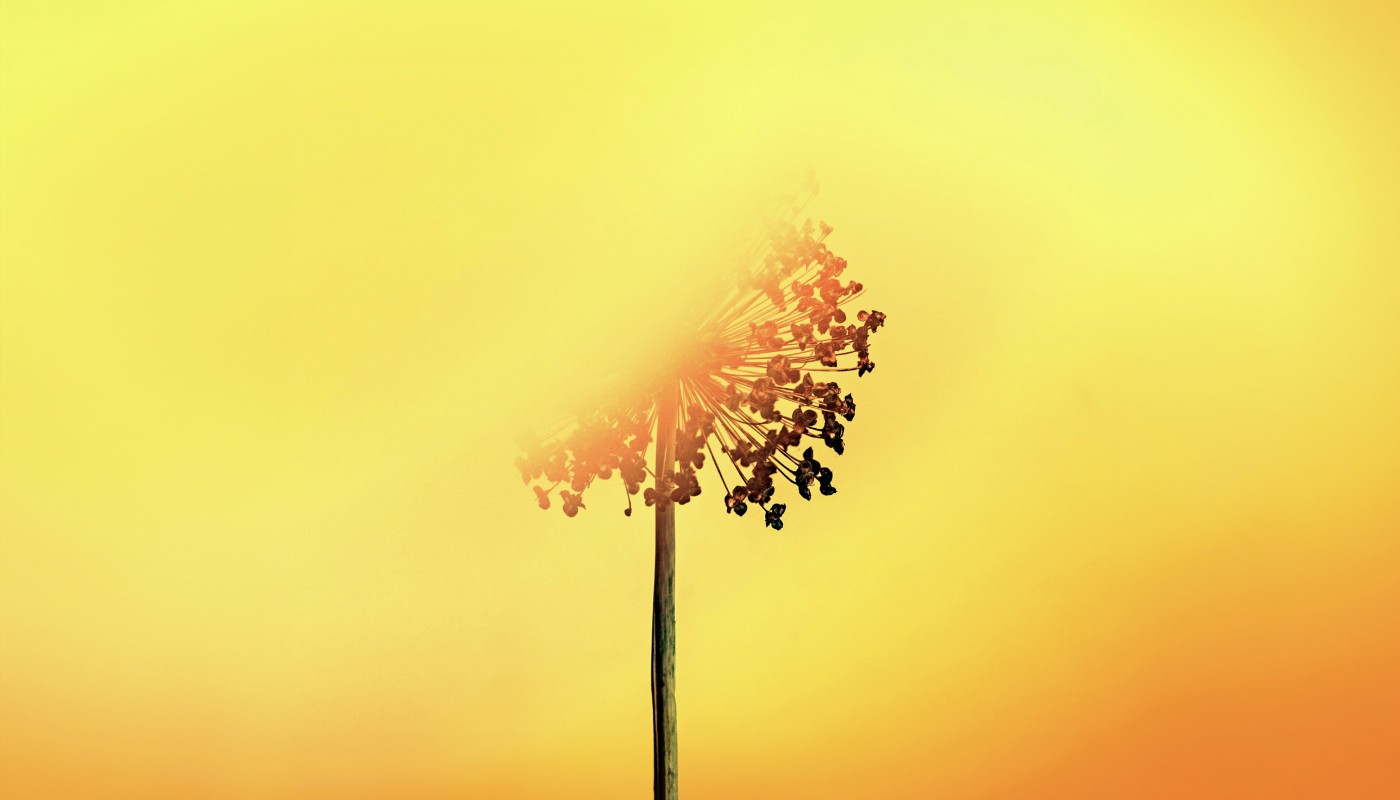
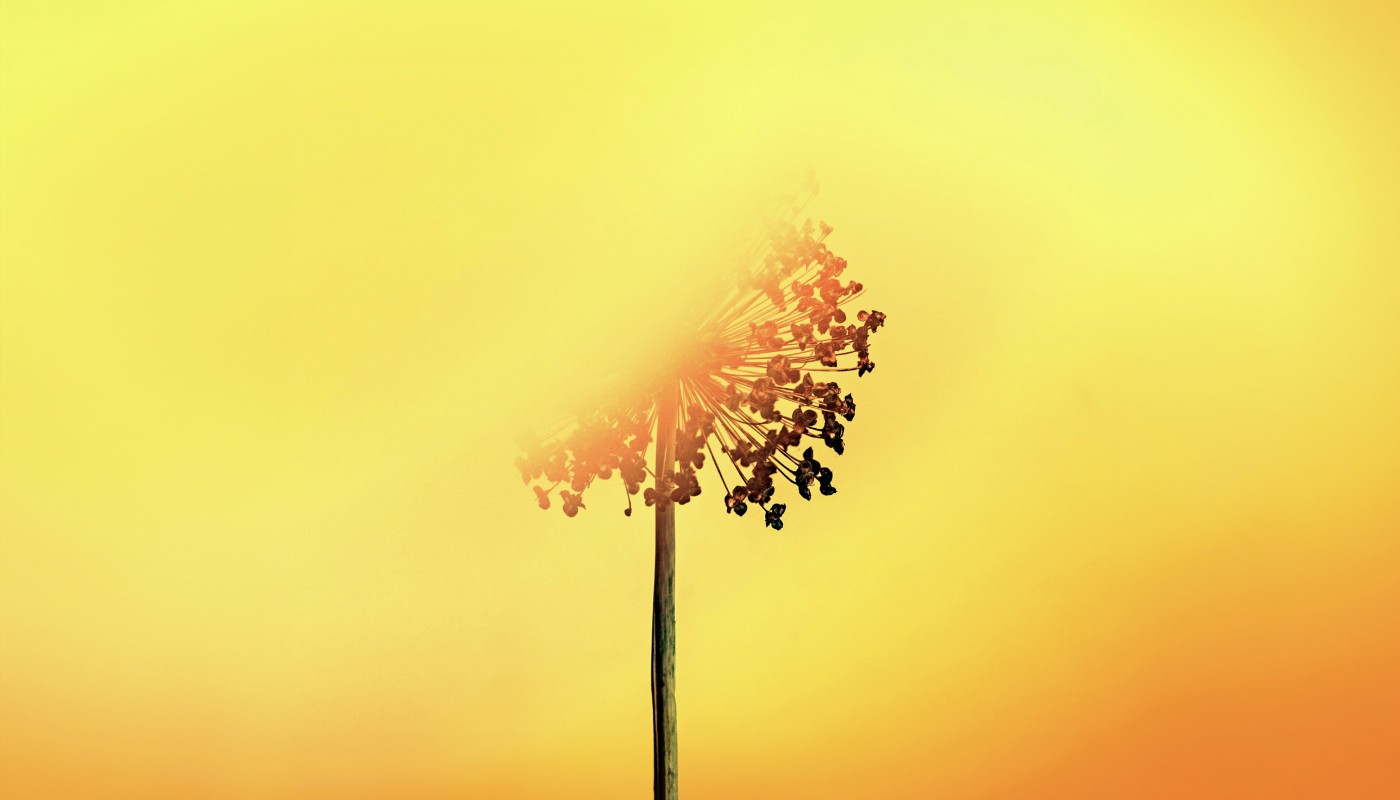
NIST’s New Quantum Cryptography Standards: What You Need to Know
Apr 25, 2025
Read our latest commentary and research on the post-quantum encryption space
Read our latest commentary and research on the post-quantum encryption space


Texas Quantum Initiative Passes: Lone Star State Bids to Become Quantum Powerhouse


Europe’s Quantum Surge: Bridging the Private Funding Gap for Tech Dominance


Racing the Quantum Threat: 5 Nations Compress Post-Quantum Cryptography Timelines


Microsoft’s Azure Quantum Unveils 4D Code Plan to Tame Quantum Errors


How Post‑Quantum Cryptography Could Have Stopped the $1.5 Billion Bybit Hack


IIT Delhi Achieves Quantum Breakthrough: Wireless Communication Over 1 Kilometer


Caltech Scientists Achieve Hyper-Entanglement in Atomic Motion: A Quantum Leap in Control and Coherence


Quantum Boom: Surge in Tech Deals & Funding Marks a Turning Point in 2025


Pasqal Charts Bold Course: Roadmap to 10,000 Qubits and Fault-Tolerant Quantum Computing


Quantum at a Turning Point: Nvidia CEO Declares Industry at ‘Inflection Point’


IBM Unveils Next-Generation Quantum Processor, Ushering In a New Era of Computation


IonQ Acquires Oxford Ionics for $1.08 Billion: A Bold Leap Toward Fault‑Tolerant Quantum Computing


Post‑Quantum Cryptography Takes Center Stage at Infosecurity Europe 2025


Oxford Quantum Circuits Unveils Bold Roadmap to 50,000 Logical Qubits by 2034


Caltech Achieves Hyper-Entanglement: A Quantum Breakthrough with Major Implications

Texas Quantum Initiative Passes: Lone Star State Bids to Become Quantum Powerhouse

Europe’s Quantum Surge: Bridging the Private Funding Gap for Tech Dominance

Racing the Quantum Threat: 5 Nations Compress Post-Quantum Cryptography Timelines

Microsoft’s Azure Quantum Unveils 4D Code Plan to Tame Quantum Errors

How Post‑Quantum Cryptography Could Have Stopped the $1.5 Billion Bybit Hack

IIT Delhi Achieves Quantum Breakthrough: Wireless Communication Over 1 Kilometer

Caltech Scientists Achieve Hyper-Entanglement in Atomic Motion: A Quantum Leap in Control and Coherence

Quantum Boom: Surge in Tech Deals & Funding Marks a Turning Point in 2025

Pasqal Charts Bold Course: Roadmap to 10,000 Qubits and Fault-Tolerant Quantum Computing

Quantum at a Turning Point: Nvidia CEO Declares Industry at ‘Inflection Point’

IBM Unveils Next-Generation Quantum Processor, Ushering In a New Era of Computation

IonQ Acquires Oxford Ionics for $1.08 Billion: A Bold Leap Toward Fault‑Tolerant Quantum Computing

Post‑Quantum Cryptography Takes Center Stage at Infosecurity Europe 2025

Oxford Quantum Circuits Unveils Bold Roadmap to 50,000 Logical Qubits by 2034

Caltech Achieves Hyper-Entanglement: A Quantum Breakthrough with Major Implications
In a major milestone for quantum computing, researchers Humberto Muñoz-Bauza and Daniel Lidar have demonstrated the first clear scaling advantage of quantum annealing in approximate optimization—a critical task in fields like logistics, finance, and machine learning. Published in Physical Review Letters, the study reveals that quantum annealing can outperform the best-known classical heuristic method in solving complex optimization problems, provided certain conditions are met.
The Challenge: Optimization at Scale
Many real-world problems—from routing delivery trucks to portfolio optimization—boil down to finding the best configuration among a massive number of possibilities. These problems often involve “spin glasses,” which are notoriously difficult to solve. Quantum annealing, a method that leverages quantum mechanics to search for low-energy (optimal) configurations, has long been a candidate for outperforming classical algorithms in these scenarios.
But until now, no study had definitively shown a scaling advantage—meaning that as problem size increases, quantum annealers solve problems more efficiently than classical methods in a measurable way.
Quantum Annealing Correction (QAC): A Key Innovation
The study’s breakthrough lies in the use of Quantum Annealing Correction (QAC), a method that applies error suppression by embedding error-correcting codes directly into the quantum annealer's architecture. QAC leverages a majority-voting scheme among physical qubits to protect logical qubit states, effectively reducing the impact of hardware noise and analog errors.
Using QAC, the researchers implemented a highly error-tolerant system on the D-Wave Advantage quantum annealer. They tested it on large-scale spin-glass problems using up to 1,322 logical, error-corrected qubits—the largest such demonstration to date.
The Benchmark: Outperforming the Best Classical Heuristic
The quantum system was pitted against parallel tempering with isoenergetic cluster moves (PT-ICM), a top-tier classical algorithm. The key metric was time-to-epsilon (TTε), which measures how quickly a solver finds solutions within a certain percentage (ε) of the optimal answer.
At an optimality gap of 1% or higher, quantum annealing with QAC consistently outscaled PT-ICM, providing faster approximate solutions as problem sizes grew.
Even without error correction (a method called C3), quantum annealers showed some advantage, though QAC clearly performed best.
This scaling advantage was not just a one-time performance win—it persisted across varying problem sizes and noise levels, and the improvement grew stronger with larger optimality gaps.
Key Findings
QAC shows better scaling than classical methods at approximate solutions (ε ≥ 1.0%), which could translate to real-world speedups in tasks like machine learning or supply chain management.
Error correction is critical—without QAC, performance drops noticeably.
Fast annealing schedules (coherent regimes) do not necessarily improve scaling and may be more susceptible to thermal errors.
Quantum tunneling is suspected to play a role in this speedup, offering a potential mechanism behind the improved performance.
Why This Matters
This research marks a turning point in quantum optimization, providing the first evidence that quantum annealers can surpass classical algorithms not just in runtime but in how they scale with increasing problem complexity.
While this is an early-stage result confined to synthetic spin-glass problems, it opens the door to applying quantum advantage in real-world use cases, particularly where high-precision, approximate answers are acceptable or even preferred.
What’s Next?
The next challenge is to:
Extend these findings to more complex, application-specific problems with dense connectivity.
Optimize hardware schedules and error-suppression techniques for broader use.
Continue refining metrics like TTε to evaluate future quantum and hybrid solvers.
Bottom Line:
Quantum computing has taken a significant leap from theory to tangible performance gains. Thanks to innovations like QAC and improved benchmarking, we now have credible evidence that quantum annealing isn’t just a niche tool—but a competitive and scalable approach for solving some of the world’s hardest computational problems.
Citation: Muñoz-Bauza, H., & Lidar, D. (2025). Scaling Advantage in Approximate Optimization with Quantum Annealing. Physical Review Letters, 134(16), 160601. https://doi.org/10.1103/PhysRevLett.134.160601
Let's talk!
Office:
1535 Broadway
New York, NY 10036
USA
Local time:
17:20:05
Let's talk!
Office:
1535 Broadway
New York, NY 10036
USA
Local time:
17:20:05