Advancing Quantum Machine Learning with Multi-Chip Ensemble Architectures
Advancing Quantum Machine Learning with Multi-Chip Ensemble Architectures
May 14, 2025
Quantum Machine Learning (QML) holds immense promise for tackling complex problems across various domains, from healthcare to finance. However, the practical deployment of QML is hindered by the limitations of current Noisy Intermediate-Scale Quantum (NISQ) devices, which include issues like noise, limited scalability, and training difficulties in variational quantum circuits (VQCs). A novel approach, the Multi-Chip Ensemble VQC framework, offers a compelling solution to these challenges.
The Multi-Chip Ensemble VQC Framework
Developed by researchers including Junghoon Justin Park, Jiook Cha, Samuel Yen-Chi Chen, Huan-Hsin Tseng, and Shinjae Yoo, the Multi-Chip Ensemble VQC framework partitions high-dimensional quantum computations across multiple smaller quantum chips. Each chip processes a subset of the data, and their outputs are aggregated classically. This modular approach enhances scalability and resilience to noise, addressing key limitations of NISQ devices.
Key benefits of this framework include:
Scalability: By distributing computations, the system can handle larger problems without requiring a single, large-scale quantum processor.
Noise Resilience: Smaller circuits on individual chips are less susceptible to errors, and aggregating results helps average out noise.
Improved Trainability: The framework mitigates the "barren plateau" problem—where gradients vanish during training—by maintaining effective gradients across the ensemble.
The researchers validated the framework using standard datasets such as MNIST, FashionMNIST, CIFAR-10, and the real-world PhysioNet EEG dataset. Simulations under realistic noise models, including depolarizing and amplitude-damping noise, demonstrated the framework's robustness and potential for practical QML applications.
Alignment with Industry Trends
The Multi-Chip Ensemble VQC framework aligns with broader industry efforts to develop scalable quantum architectures. For instance, Rigetti Computing has introduced a modular, multi-chip quantum processor that connects multiple identical dies into a large-scale processor, facilitating scalability and reducing manufacturing complexity. Similarly, researchers at MIT have developed a modular hardware platform integrating thousands of interconnected qubits onto a customized integrated circuit, enabling precise control and scalability.
These developments underscore a shift towards modular and scalable quantum computing solutions, which are essential for advancing QML and other quantum applications.
Conclusion
The Multi-Chip Ensemble VQC framework represents a significant step forward in making Quantum Machine Learning more practical and scalable. By leveraging a modular approach that distributes computations across multiple chips, the framework addresses key challenges of NISQ devices, including noise and limited scalability. Its compatibility with current and emerging quantum hardware architectures positions it as a promising solution for advancing QML in the near term.
As the quantum computing industry continues to evolve, approaches like the Multi-Chip Ensemble VQC framework will be crucial in bridging the gap between theoretical potential and practical application, bringing us closer to realizing the full benefits of quantum technologies.
Reference:
Park, J. J., Cha, J., Chen, S. Y.-C., Tseng, H.-H., & Yoo, S. (2024). Addressing the Current Challenges of Quantum Machine Learning through Multi-Chip Ensembles. arXiv preprint [arXiv:2505.08782]. https://arxiv.org/abs/2505.08782
May 14, 2025
Quantum Insights



Cracking RSA with Fewer Qubits: What Google's New Quantum Factoring Estimate Means for Cybersecurity
May 28, 2025



Quantum Arms Race: U.S. Defense Intelligence Flags Rivals’ Growing Military Use of Quantum Tech
May 27, 2025



Quantum Threats and Bitcoin: Why BlackRock’s Warning Matters for the Future of Crypto Security
May 27, 2025



Sudbury's SNOLAB Ventures into Quantum Computing Research
May 26, 2025



Lockheed Martin and IBM Pioneer Quantum-Classical Hybrid Computing for Complex Molecular Simulations
May 23, 2025



Why the Moon Matters for Quantum Computing: From Helium-3 to Off-Planet Quantum Networks
May 23, 2025



NIST Approves Three Post-Quantum Cryptography Standards: A Milestone for Digital Security
May 22, 2025



Scientists Connect Quantum Processors via Fiber Optic Cable for the First Time
May 21, 2025



Quantum Computing and Encryption Breakthroughs in 2025: A New Era of Innovation
May 21, 2025



How CISOs Can Defend Against the “Harvest Now, Decrypt Later” Threat
May 20, 2025



NVIDIA Expands Quantum and AI Ecosystem in Taiwan Through Strategic Partnerships and Supercomputing Initiatives
May 19, 2025



Quantum Annealing Breakthrough: Quantum Computer Outperforms Fastest Supercomputers
May 18, 2025



Quantum Computing's New Frontier: How the $1.4 Trillion US–UAE Investment Deal is Shaping the Industry
May 16, 2025



Quantum Computing Meets Cancer Research: A New Frontier in Drug Discovery
May 16, 2025



Quantum Industry Leaders Urge Congress to Reauthorize and Expand National Quantum Initiative
May 15, 2025



Honeywell's Quantinuum and Qatar's Al Rabban Capital Forge $1 Billion Quantum Computing Joint Venture
May 15, 2025



Advancing Quantum Machine Learning with Multi-Chip Ensemble Architectures
May 14, 2025



How will the new US-Saudi Arabia AI deal effect the Quantum Computing industry?
May 14, 2025



Saudi Arabia's $600 Billion AI Push: Amazon, Nvidia, and Global Tech Giants Lead the Charge
May 14, 2025



Quantum Computing Breakthrough: Diamond Qubits Achieve Unprecedented Precision
Apr 28, 2025



Australia’s Quantum Cryptography Roadmap: Preparing for a Post-Quantum Future
Apr 26, 2025
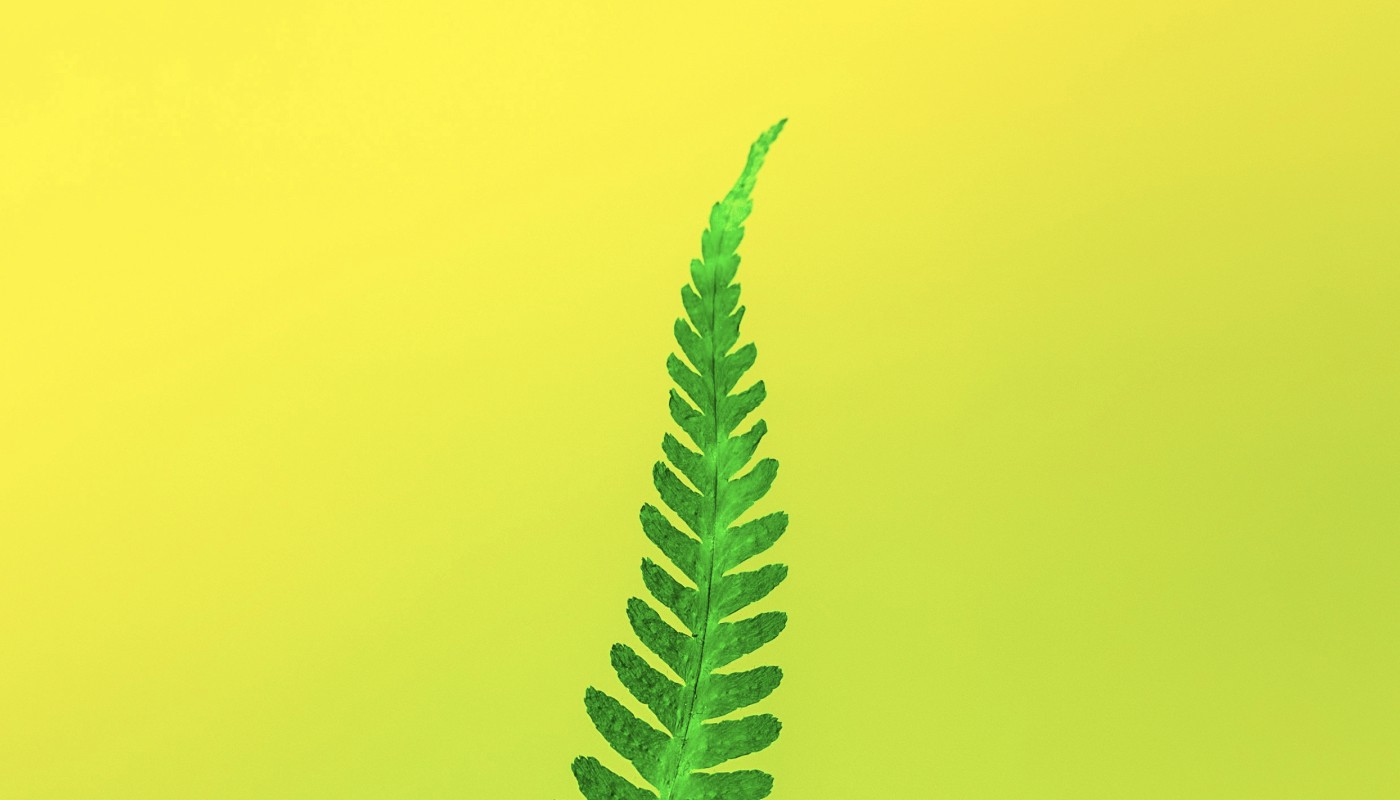
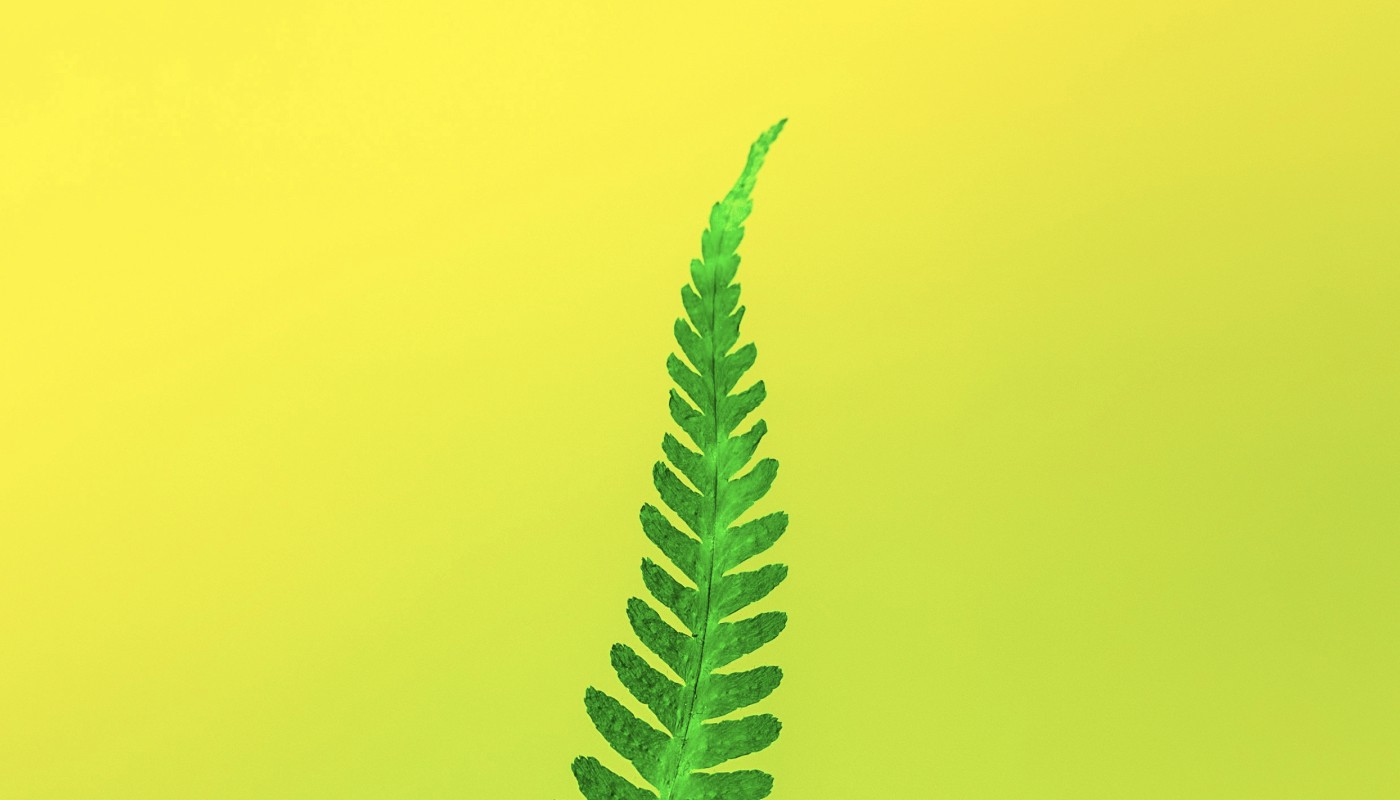
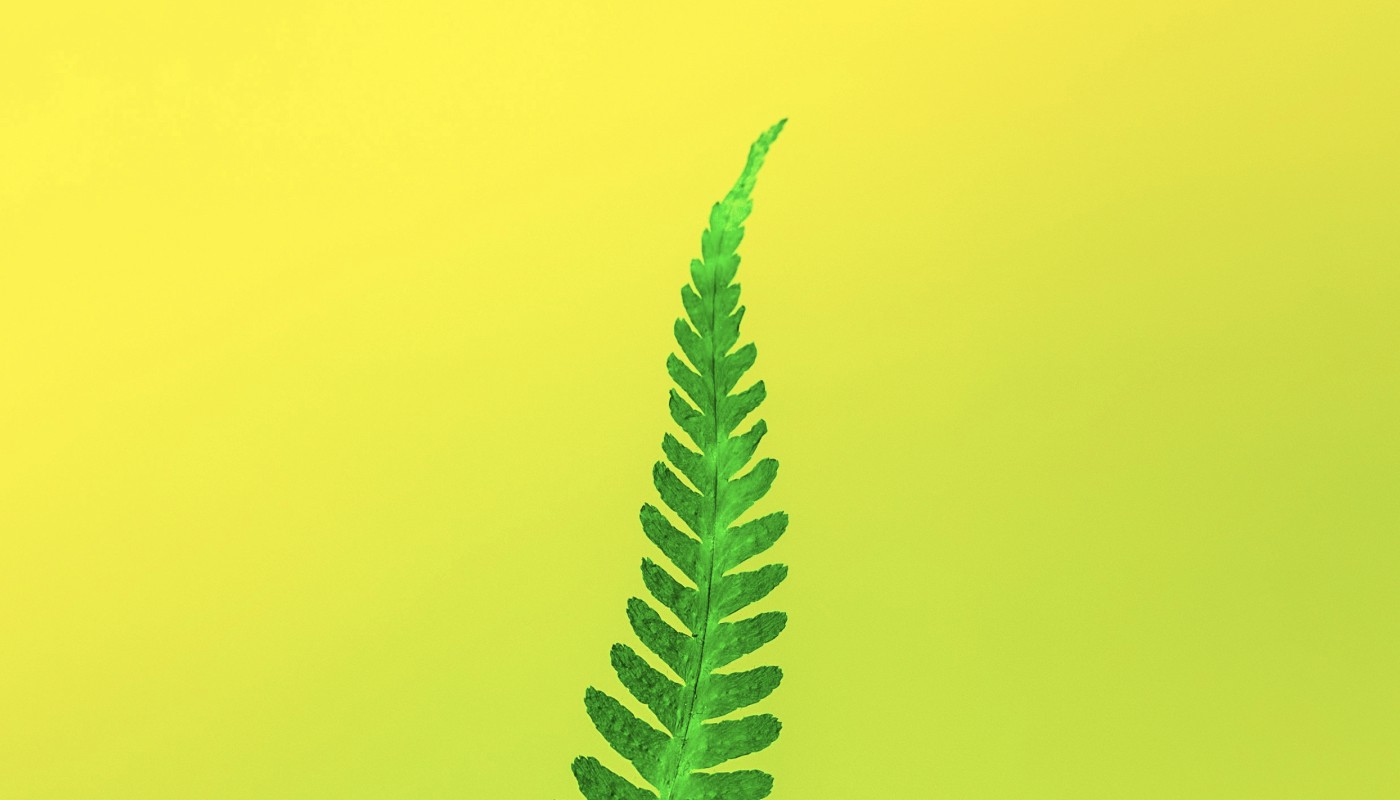
Harvest Now, Decrypt later
Apr 25, 2025
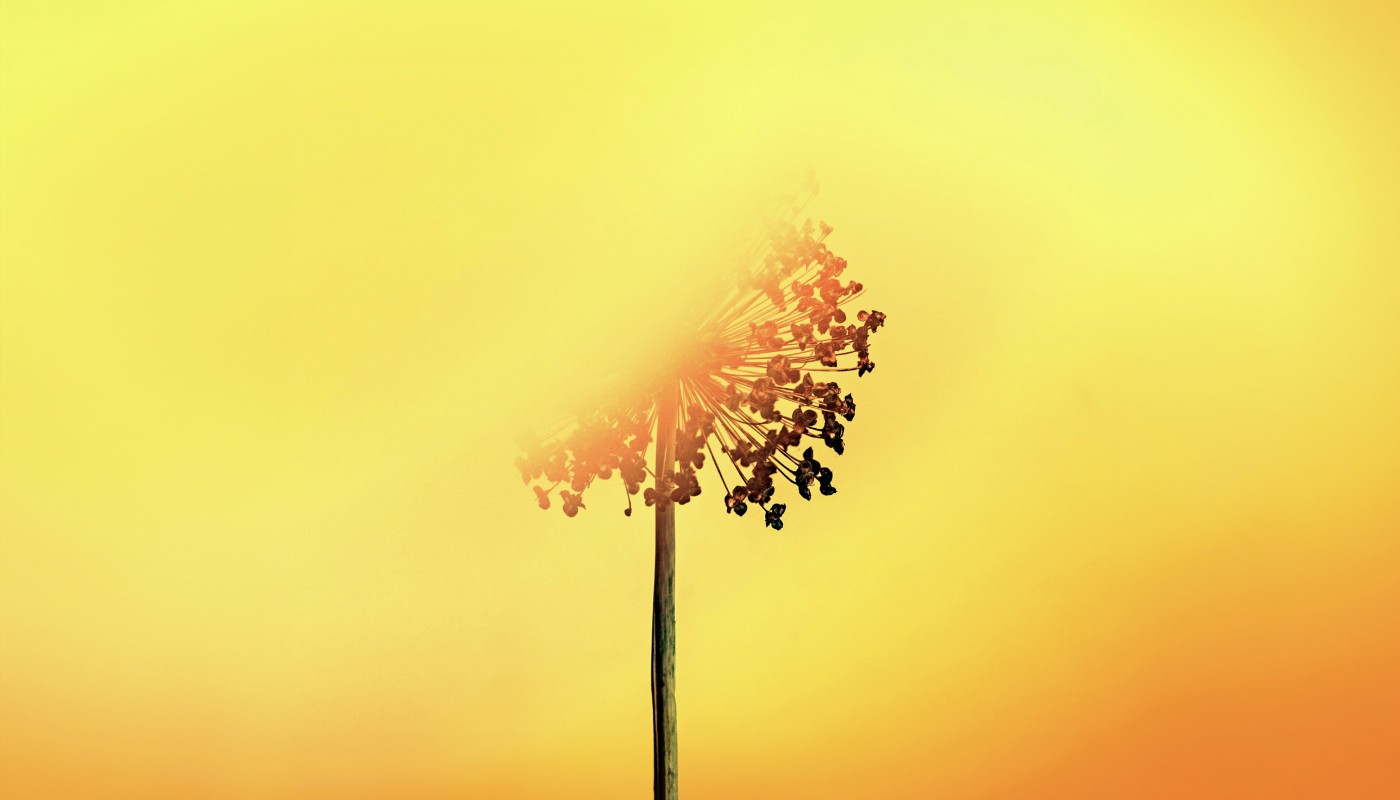
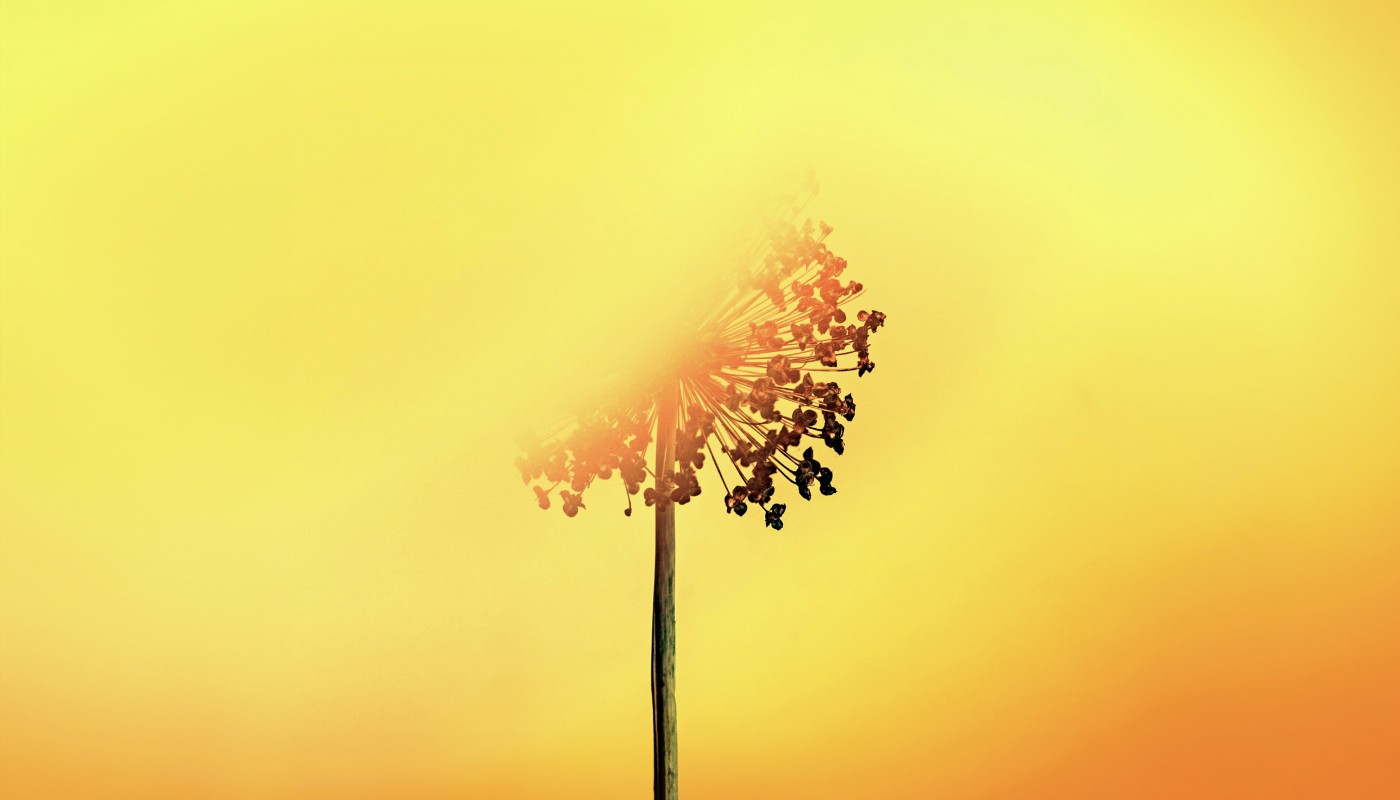
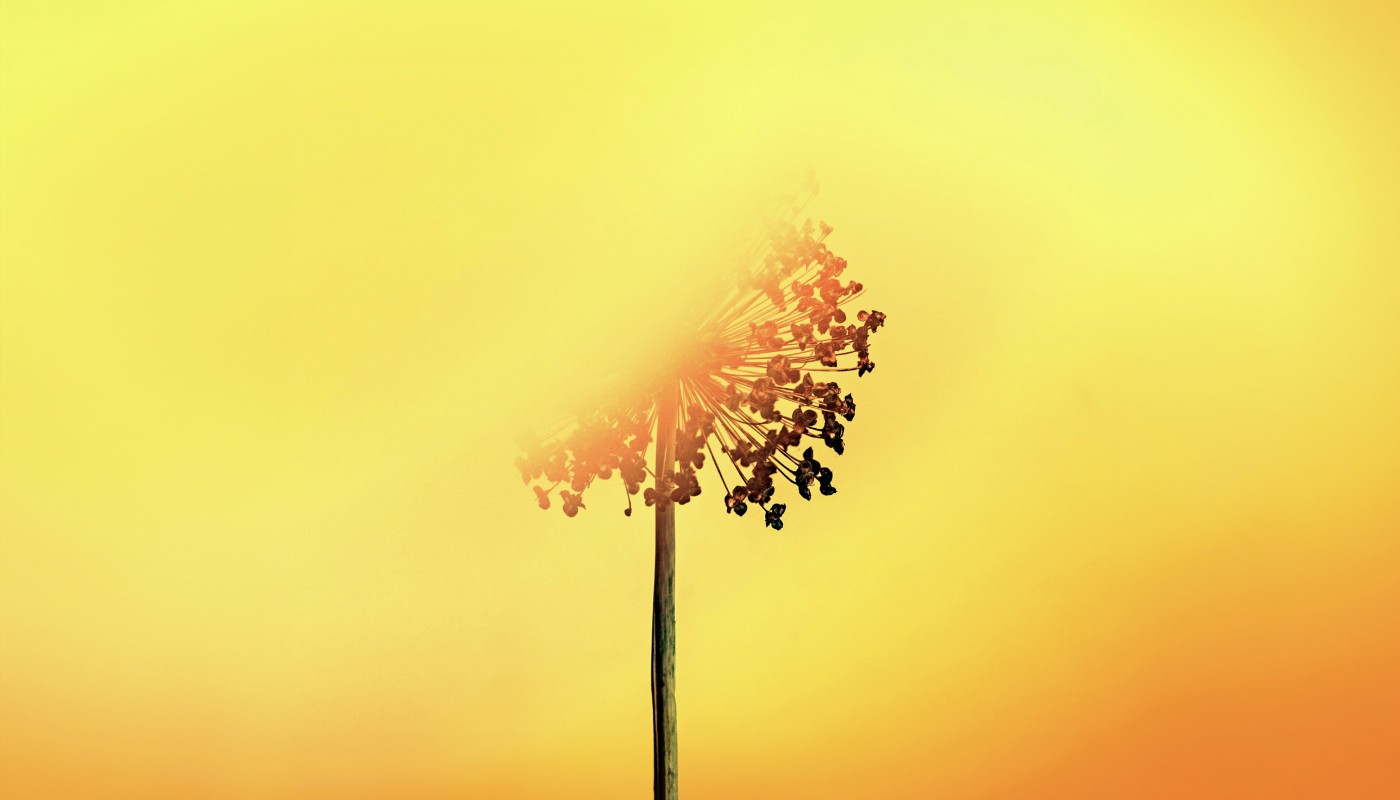
NIST’s New Quantum Cryptography Standards: What You Need to Know
Apr 25, 2025
Read our latest commentary and research on the post-quantum encryption space
Read our latest commentary and research on the post-quantum encryption space


Cracking RSA with Fewer Qubits: What Google's New Quantum Factoring Estimate Means for Cybersecurity


Quantum Arms Race: U.S. Defense Intelligence Flags Rivals’ Growing Military Use of Quantum Tech


Quantum Threats and Bitcoin: Why BlackRock’s Warning Matters for the Future of Crypto Security


Sudbury's SNOLAB Ventures into Quantum Computing Research


Lockheed Martin and IBM Pioneer Quantum-Classical Hybrid Computing for Complex Molecular Simulations


Why the Moon Matters for Quantum Computing: From Helium-3 to Off-Planet Quantum Networks


NIST Approves Three Post-Quantum Cryptography Standards: A Milestone for Digital Security


Scientists Connect Quantum Processors via Fiber Optic Cable for the First Time


Quantum Computing and Encryption Breakthroughs in 2025: A New Era of Innovation


How CISOs Can Defend Against the “Harvest Now, Decrypt Later” Threat


NVIDIA Expands Quantum and AI Ecosystem in Taiwan Through Strategic Partnerships and Supercomputing Initiatives


Quantum Annealing Breakthrough: Quantum Computer Outperforms Fastest Supercomputers

Cracking RSA with Fewer Qubits: What Google's New Quantum Factoring Estimate Means for Cybersecurity

Quantum Arms Race: U.S. Defense Intelligence Flags Rivals’ Growing Military Use of Quantum Tech

Quantum Threats and Bitcoin: Why BlackRock’s Warning Matters for the Future of Crypto Security

Sudbury's SNOLAB Ventures into Quantum Computing Research

Lockheed Martin and IBM Pioneer Quantum-Classical Hybrid Computing for Complex Molecular Simulations

Why the Moon Matters for Quantum Computing: From Helium-3 to Off-Planet Quantum Networks

NIST Approves Three Post-Quantum Cryptography Standards: A Milestone for Digital Security

Scientists Connect Quantum Processors via Fiber Optic Cable for the First Time

Quantum Computing and Encryption Breakthroughs in 2025: A New Era of Innovation

How CISOs Can Defend Against the “Harvest Now, Decrypt Later” Threat

NVIDIA Expands Quantum and AI Ecosystem in Taiwan Through Strategic Partnerships and Supercomputing Initiatives

Quantum Annealing Breakthrough: Quantum Computer Outperforms Fastest Supercomputers

Quantum Computing's New Frontier: How the $1.4 Trillion US–UAE Investment Deal is Shaping the Industry

Quantum Computing Meets Cancer Research: A New Frontier in Drug Discovery

Quantum Industry Leaders Urge Congress to Reauthorize and Expand National Quantum Initiative
Quantum Machine Learning (QML) holds immense promise for tackling complex problems across various domains, from healthcare to finance. However, the practical deployment of QML is hindered by the limitations of current Noisy Intermediate-Scale Quantum (NISQ) devices, which include issues like noise, limited scalability, and training difficulties in variational quantum circuits (VQCs). A novel approach, the Multi-Chip Ensemble VQC framework, offers a compelling solution to these challenges.
The Multi-Chip Ensemble VQC Framework
Developed by researchers including Junghoon Justin Park, Jiook Cha, Samuel Yen-Chi Chen, Huan-Hsin Tseng, and Shinjae Yoo, the Multi-Chip Ensemble VQC framework partitions high-dimensional quantum computations across multiple smaller quantum chips. Each chip processes a subset of the data, and their outputs are aggregated classically. This modular approach enhances scalability and resilience to noise, addressing key limitations of NISQ devices.
Key benefits of this framework include:
Scalability: By distributing computations, the system can handle larger problems without requiring a single, large-scale quantum processor.
Noise Resilience: Smaller circuits on individual chips are less susceptible to errors, and aggregating results helps average out noise.
Improved Trainability: The framework mitigates the "barren plateau" problem—where gradients vanish during training—by maintaining effective gradients across the ensemble.
The researchers validated the framework using standard datasets such as MNIST, FashionMNIST, CIFAR-10, and the real-world PhysioNet EEG dataset. Simulations under realistic noise models, including depolarizing and amplitude-damping noise, demonstrated the framework's robustness and potential for practical QML applications.
Alignment with Industry Trends
The Multi-Chip Ensemble VQC framework aligns with broader industry efforts to develop scalable quantum architectures. For instance, Rigetti Computing has introduced a modular, multi-chip quantum processor that connects multiple identical dies into a large-scale processor, facilitating scalability and reducing manufacturing complexity. Similarly, researchers at MIT have developed a modular hardware platform integrating thousands of interconnected qubits onto a customized integrated circuit, enabling precise control and scalability.
These developments underscore a shift towards modular and scalable quantum computing solutions, which are essential for advancing QML and other quantum applications.
Conclusion
The Multi-Chip Ensemble VQC framework represents a significant step forward in making Quantum Machine Learning more practical and scalable. By leveraging a modular approach that distributes computations across multiple chips, the framework addresses key challenges of NISQ devices, including noise and limited scalability. Its compatibility with current and emerging quantum hardware architectures positions it as a promising solution for advancing QML in the near term.
As the quantum computing industry continues to evolve, approaches like the Multi-Chip Ensemble VQC framework will be crucial in bridging the gap between theoretical potential and practical application, bringing us closer to realizing the full benefits of quantum technologies.
Reference:
Park, J. J., Cha, J., Chen, S. Y.-C., Tseng, H.-H., & Yoo, S. (2024). Addressing the Current Challenges of Quantum Machine Learning through Multi-Chip Ensembles. arXiv preprint [arXiv:2505.08782]. https://arxiv.org/abs/2505.08782
Let's talk!
Office:
1535 Broadway
New York, NY 10036
USA
Local time:
20:13:00
Let's talk!
Office:
1535 Broadway
New York, NY 10036
USA
Local time:
20:13:00